Enhance the shopping experience with personalized product suggestions.
Boost average order values and revenue with upsell and cross-sell promotions.
Retain customers and drive repeat business with targeted experiences.
Take advantage of collaborative filtering
A recommendation technique that predicts a user's interests by collecting preferences from a community. This method identifies patterns amongst users who share similar behaviors or preferences to make recommendations.
Collaborative Filtering leverages the "wisdom of the crowd" to provide relevant suggestions.
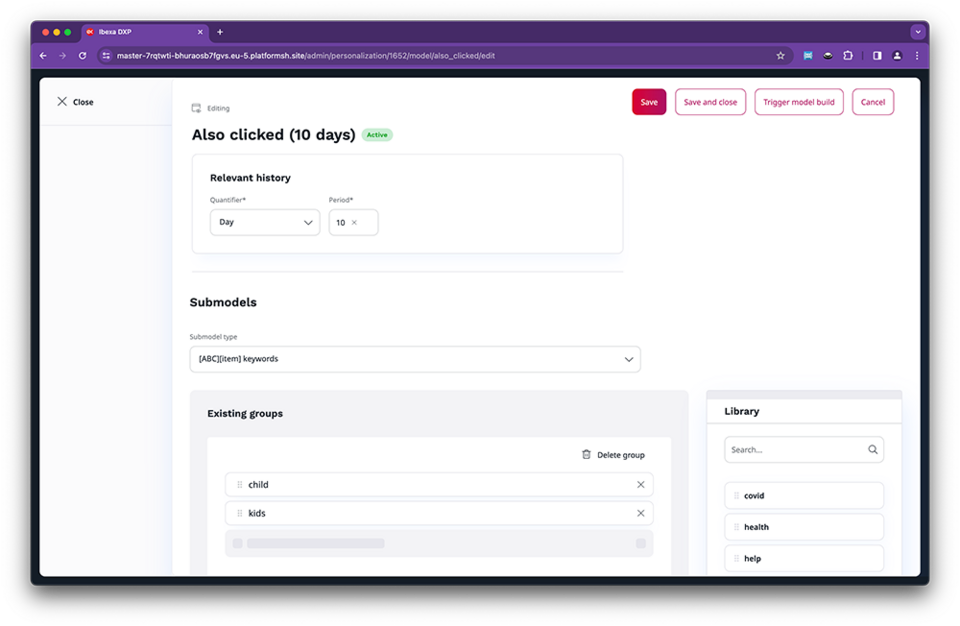
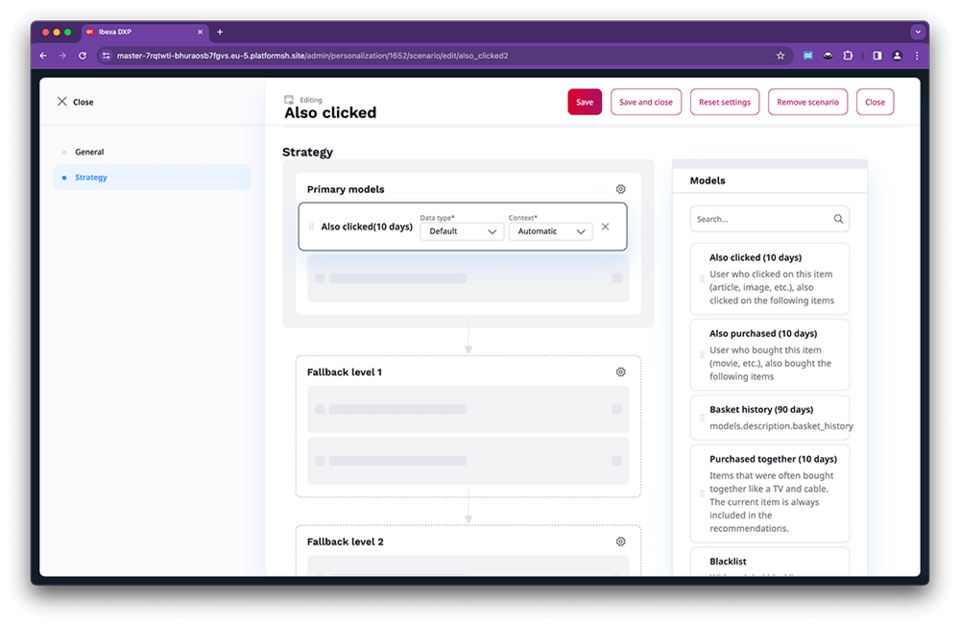
Reduce content clutter and increase engagement
With Ibexa Personalization, its possible to examine the attributes of items and compare them to a user's past behavior in order to provide recommendations that match their preferences.
This allows you to reduce the amount of "content clutter" and display only the most relevant information to increase engagement and conversion rates with a particular individual or user segment.
Succeed with personalized recommendations
Data-driven recommendations that provide tailored suggestions to individuals or segments are proven to increase the time that users spend on your platforms and the number of pages they view.
This strategy allows businesses to stand out against their competitors by delivering unique experiences, increasing the likelihood of conversions and customer retention.
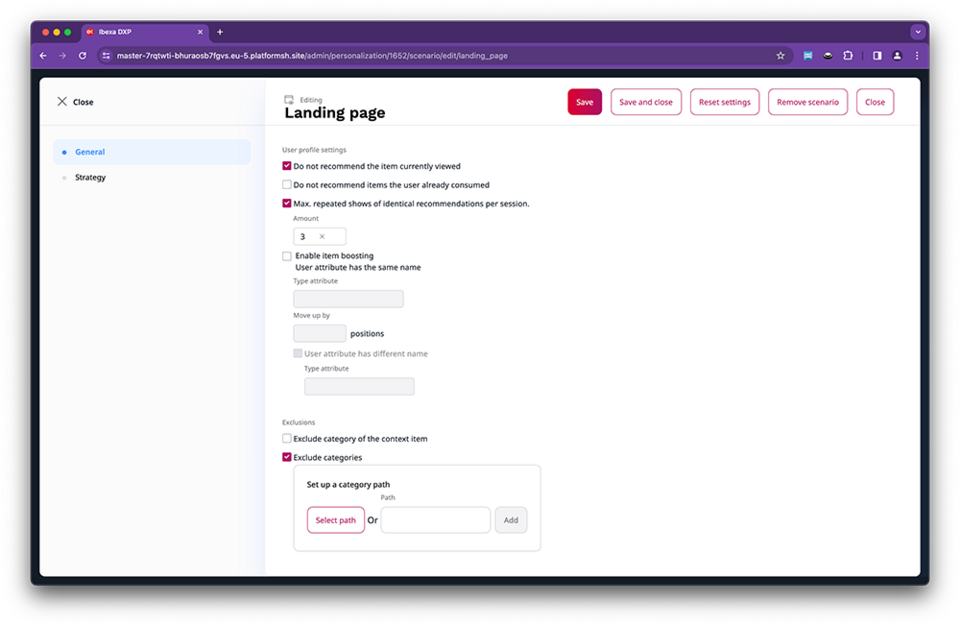
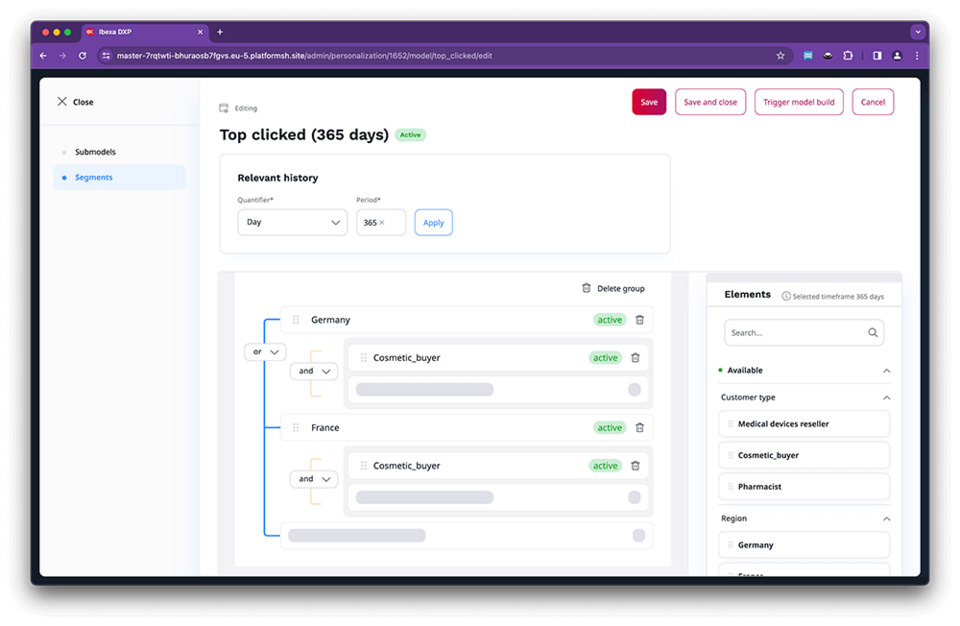
Segment visitors by attributes and behavior
Not only can you integrate user data from existing tools and platforms but you can also collect and aggregate additional information. Businesses can then group prospects and customers into specific segments based on similar demographics, interests, or behaviors.
This allows companies to deliver more precise content recommendations which are aligned to each unique user or group.
Intelligent learning for tailored recommendations
Ibexa Personalization utilizes advanced machine learning algorithms to continuously learn from user behavior, preferences, and contextual data to create comprehensive user profiles.
The algorithm learns patterns and relationships within the data that are used to predict what item a user might be interested in to improve the accuracy of recommendations.
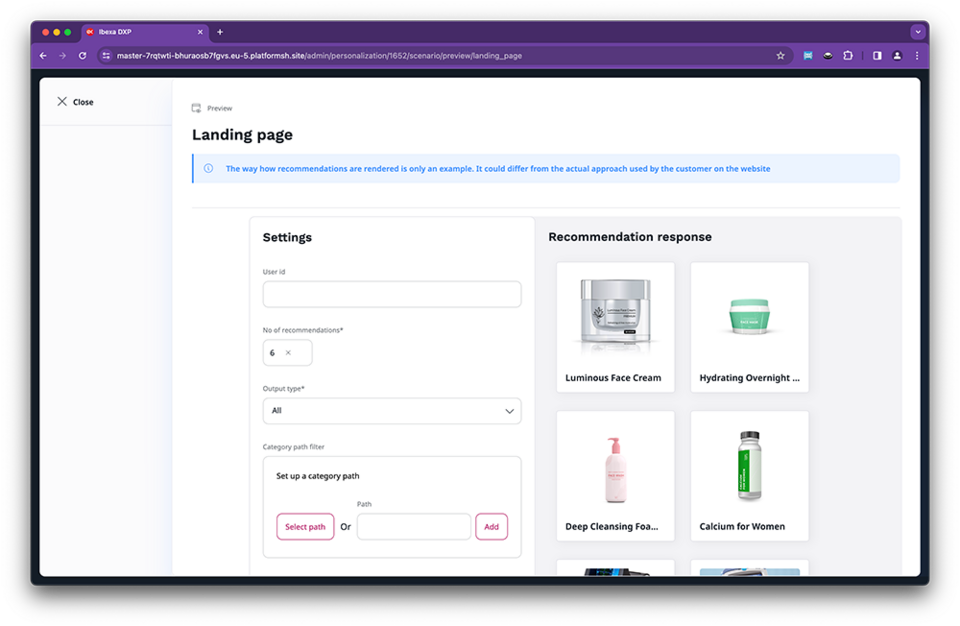
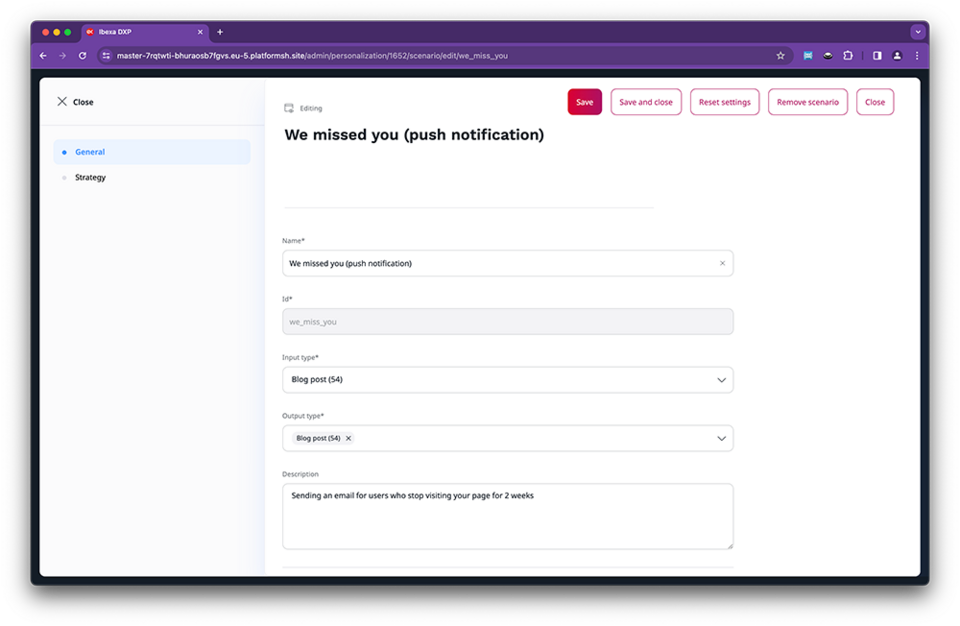
Work smarter with automated email triggers
Send targeted and personalized messages based on specific user actions, preferences, or behaviors. This enhances user engagement by delivering content that is relevant to each recipient.
Ibexa DXP is shipped with several default triggers (such as abandoned basket trigger, 'We miss you' trigger, Price drop trigger, etc) that helps you to automate specific actions (like the sending process), saving time and resources. Once set up, these triggers can work in the background, ensuring consistent communication without manual intervention.
Discover why our customers love Ibexa DXP
Discover all the different ways our customers are using Ibexa DXP to centralize, simplify, automate and create impressive digital experiences.
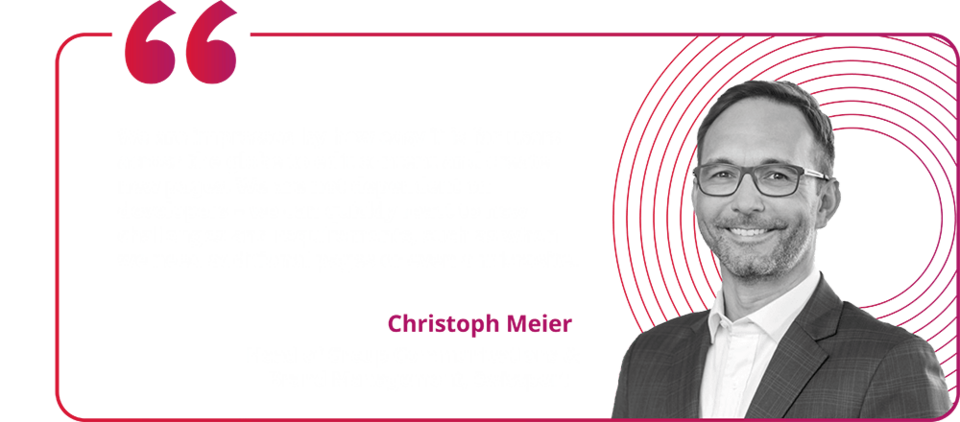
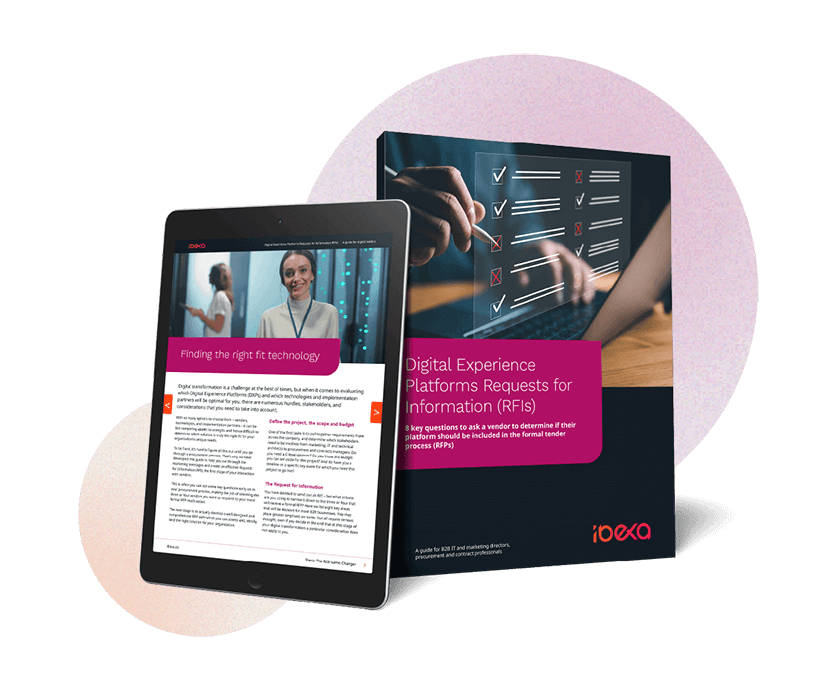
Download our free RFI eBook to help you choose the right DXP
In this free and exclusive eBook, we guide you through the process of requesting thr right information for your specific needs with a range of useful templates and information to kick-start and accelerate your digital transformation strategy.